Wind tunnel testing is an important part of aerospace manufacturing. The process involves high-speed winds being directed at aircraft components in a tunnel to observe how they would perform when in flight. This well-established method of testing is not without its drawbacks. It’s expensive and time consuming.
With this in mind, BAE Systems, in collaboration with the University of Manchester, looked to challenge traditional processes by exploring the innovative use of artificial intelligence and data processing. The resulting research programme focused on developing AI systems that can simulate different aerodynamic testing models – greatly improving efficiency and reducing costs and product development time.
Essential to Aerodynamics
Traditional wind tunnel test scenarios are carried out by passing air at high speeds across wood or metal aircraft models or individual parts in a physical tunnel in order to analyse their aerodynamic performance.
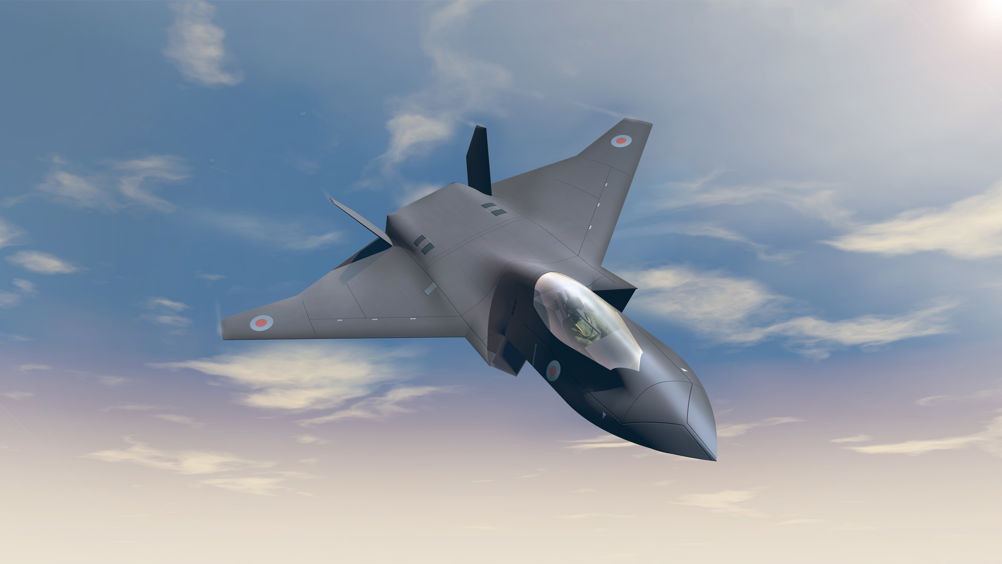
Such wind tunnel simulations can help us understand how aircraft designs will perform in real world scenarios - namely how the product in question will be impacted by aerodynamic forces and the flow of air. This helps manufacturers determine what changes need to be made as they move from virtual to real-life environments.
Wind tunnel testing is essential to all aviation manufacturing processes. Not only does it ensure aircraft are achieving peak performance but also that they’re safety compliant. However, replicating the authentic, real-life conditions that an aircraft will experience in flight uses a considerable amount of energy and, with that, there is of course a price to pay from a financial and environmental perspective. Although this kind of testing will always be needed, there are now efficiencies to be made thanks to emerging technological advances.
Next Generation Wind Tunnel Testing
That’s where AI comes into the picture. AI techniques are being explored and developed across academia. The University of Manchester is a leader in the field so we’ve worked with them to train an AI deep learning model on data from millions of historic wind tunnel tests. This allowed it to ‘learn’ how to gauge the way in which air moves at different speeds across varying aircraft panels.
What has been key to factor at the heart of this research is the need to ensure the same levels of quality control in comparison to existing or legacy aerodynamic testing methods. As part of this project, we therefore also used x-rays to allow us to check the most important components of the aircraft down to a molecular level and monitor how they react under those conditions.
As you would imagine, this technique produces a vast amount of data when checking an entire part, such as a blade in a jet turbine. Again, this historically required manual intervention for physical checking throughout the manufacturing process, but thanks to AI techniques we can now automate the evaluation stage, rapidly improving the speed and even the accuracy of results. We are now working to understand how we can further exploit this research and how we can work with regulators and customers to validate and embed any new processes.
Key Benefits
The main benefits of these AI-powered innovations are twofold: sustainability and efficiency. We have been able to prove that we can rapidly increase the speed in which we can go through a design cycle for aircraft and missiles. As an alternative to traditional wind tunnel testing, we don’t have to set up the physical tunnel to test and then analyse the results and check the impact of the product design. Instead, we can programme the system with the appropriate variables and see results in a matter of seconds, rather than the minutes and hours previously. This gives engineers the opportunity to explore more design options rapidly.
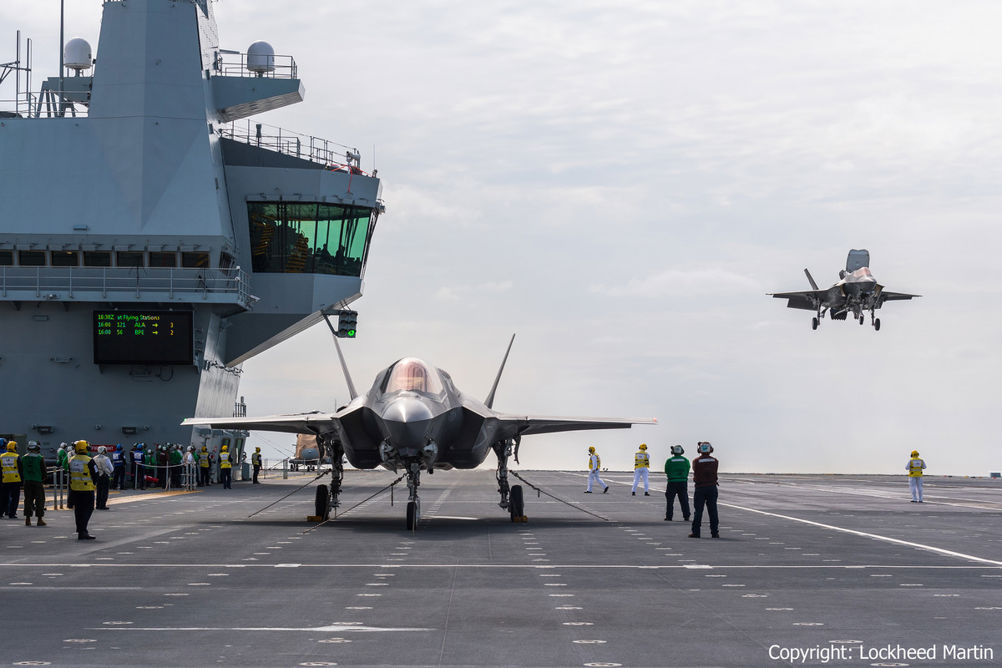
Not only does this save considerable amounts of time, but it also reduces the amount of energy we use. As previously mentioned, traditional wind tunnels use lots of energy to produce the high wind speeds required for a test. With this technology, that consumption is cut dramatically.
The Takeaways
Wind tunnel testing is paramount to aerodynamics but, with conventional methods requiring high levels of energy, BAE and the University of Manchester’s efforts are helping us be more efficient and reduce our carbon footprint considerably, paving the way for the next generation of aerodynamic testing.
The potential of AI in our work doesn’t stop there though. AI has also been used at other stages of the testing process where manual work would previously have been required for evaluation, rapidly improving the speed with which we get results and even improving their accuracy.
Thanks to the vast amounts of data that BAE Systems has access to, we can work with researchers on our most difficult data science challenges to make the best use of technological innovations, such as the development of AI, to tackle them. As we have seen with this example, the combination of our deep technical knowledge of aerospace and academia’s extensive research capability is a key way we can ensure that we make best use of emerging technologies to be more efficient and environmentally friendly, whilst not compromising on the effectiveness of our methods or the quality of our products.
Andrew Gordon is Principal Engineer for New Technology in BAE Systems’ Air business
Comment: Autonomous construction requires open data standards
The UK is particularly well served with topographic data thanks to the Environment Agency´s LIDAR programs, specifically the composite digital terrain...