Transmission electron microscopy (TEM) is a powerful imaging technique that allows us to capture images of materials even down to the atomic scale. The approach uses electromagnets, formed from coils of copper wire, to control and focus the electron beam and to magnify the created signal and generate images. These microscopes have existed for almost 100 years but have evolved rapidly in the last decade. A key reason for recent progress is the increasingly cost-effective access to advanced computation, allowing precise control over the currents passing through the many hundreds of different power supplies and therefore the microscopes’ ability to generate atomic resolution images. The capability to image at the atomic scale has helped to revolutionise our understanding of materials and allowed us to use this knowledge to develop materials with improved performance.
What is needed now?
Despite the power of the TEM technique, many of the most challenging problems in materials involve complex heterogeneous systems where the approach provides an incomplete picture. As a high spatial resolution, nanoscale technique, TEM can struggle to analyse technologically relevant volumes of sample. This can raise questions about sample representativeness and drives electron microscopists to analyse model systems, which can be made with greater homogeneity. However, it often leaves a gap between the material analysis and real-world industrial applications.
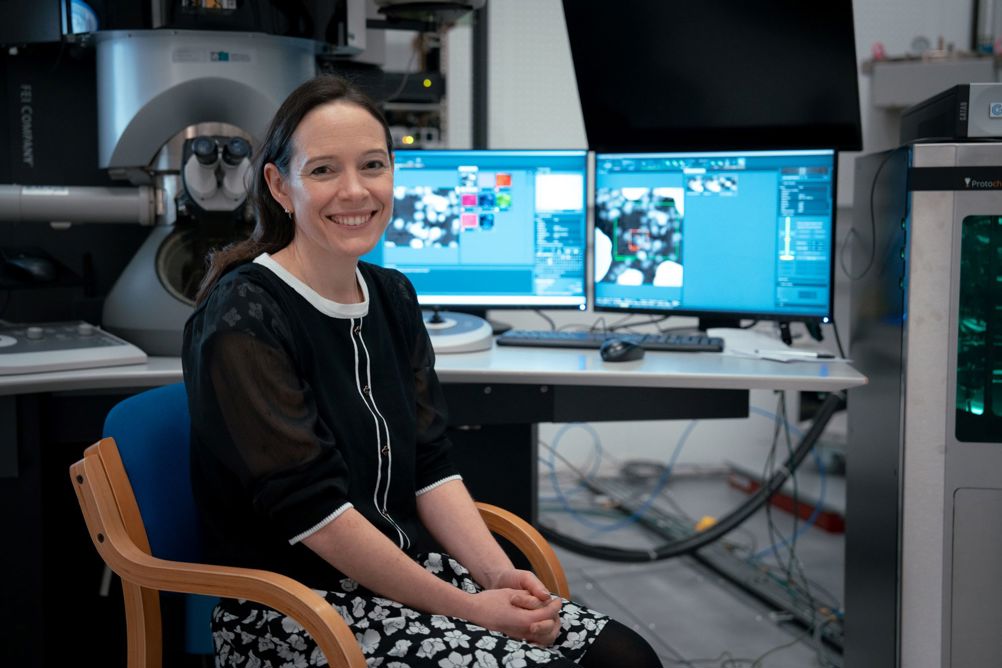
A technologically important example of this is when characterising the heterogeneous catalysts (nanoparticles on oxide) which are widely used industrially in the production of most chemical products. If we’re only able to view a small number of nanoparticles, we might miss the ones that are most important in determining why a catalyst formulation is more or less effective. The same problem can occur when characterising an engineering component, if only a handful of grain boundaries are analysed the entire material structure might not be accurately represented.
A second aspect to only analysing a small volume is that where the feature we are seeking to identify is sparsely distributed, they can be impossible to locate. If you are trying to find the sparse single atoms that are key to the function of a particular optical or electronic component then it is often too time consuming or expensive to take the millions of images needed find these manually. We aim to build a new generation of electron microscope that will address these challenges. Here’s how.
How we’re using AI and automation
Currently, we’re working with computers to expand the scope of material accessible through TEM imaging. By employing AI algorithms such as convolutional neural networks we are already able to train a computer to identify features in images much more quickly and with similar (or better) reliability than humans can. We are now using this success to get the computers to control the microscope to search for regions of the sample that contain the features we are interested in. For example, we might want the microscope to take images of all the nanoparticles within a particular size range, with a particular oxidation state or having a specific composition. Alternatively, we might want it to find an atomic dopant atom or image all grain boundaries to determine which ones have precipitates associated with them. Our objective is to establish capabilities for analysis and high-throughput data-collection, enabling a more comprehensive grasp of material behaviour.
Integrating AI and machine learning techniques into the microscope allows us to enhance the efficiency of TEM data collection, as well as to remove human bias. Yet TEM is usually a very hands-on experiment. The alignment of the system is typically checked and tweaked at hourly intervals to generate the best data. To achieve a fully automated TEM capable of atomic resolution imaging and elemental analysis with no human intervention therefore requires algorithms to also be able to perform the instrument alignments. Essentially the TEM must be able to correctly adjust itself to get the perfect image. This is now possible on the latest generation of instruments thanks to manufacturer developments in automation. We have enabled this automation to work successfully every time by developing ultra stable environmental conditions, and University of Manchester have invested more than £1m in ensuring our state of the art room for the new facility has the lowest possible noise, lowest possible mechanical vibrations as well as the most stable temperature and magnetic field conditions.
Diverse applications and benefits
The integration of AI and automation in TEM has huge potential benefits for industrial material analysis. Once trained, the instrument is many times more efficient at performing microscopy than an expert human. It does not require coffee breaks and can run independently over nights and weekends. This gives the potential for cost and efficiency savings, as well as for generating more powerful microstructural data sets. This new analysis capability has great benefit for the study of heterogeneous particulate systems and engineering alloys, where we require analysis of multiple data points for a full understanding of the materials.
We believe this technology will also prove crucial in the development of quantum materials, where precise imaging and analysis of atomic structures and defects are essential. There are also synergies and potential to impact areas of biological electron microscopy and healthcare research, where the strength would similarly come from an ability to automatically identify and zoom in on features that need more detailed study.
With automated data-collection capabilities, we’re able to reduce human limitations and improve data accuracy. It also opens the door for us to analyse large datasets such as elemental maps or oxidation states more quickly – again giving us the opportunity to gain a far more comprehensive understanding of material properties. Flexibility is also key: training computers to adapt to different material types and applications has allowed us to transition between imaging tasks far quicker.
Shaping the future of material analysis
Looking ahead, we anticipate further advancements in TEM technology, including improvements in detector efficiency, stability and automated alignment systems, will allow us to continue to push the envelope over the range of materials for which we are able to achieve extremely precise atomic resolution imaging. All of which makes the potential for widespread use of AI-assisted TEM in industrial and research settings, huge.
As an example of how we’re using TEM techniques to solve real-world problems, we’re collaborating with industry partners such as bp in areas such as developing sustainable fuels from municipal waste.
This is where higher education institutions, like the University of Manchester, play a vital role: we’re driving forward research and innovation and working with manufacturers to address industrial challenges.
A revolutionary approach to material understanding
The integration of AI and automation in transmission electron microscopy is revolutionising material analysis and research. By increasing data throughput, accuracy, and efficiency, we can gain a deeper understanding of the atomic structures and properties of materials, unlocking new possibilities in fields ranging from industrial catalysis to quantum material development.
As we continue to push the boundaries of this technology, collaborations between academia and industry will be essential in driving innovation and addressing real-world challenges.
Professor Sarah Haigh is Director of the Electron Microscopy Centre (EMC) at The University of Manchester
Industry welcomes incoming Labour government
Could you do a survey of engineers to see how many believe that the Labour policy to decarbonise electricity generation by 2030 is possible?