Engineering is the fusion of science and human creativity. It thrives on new ideas. But how much of an engineer’s time is actually spent creating or refining those ideas?
Many hours can be lost in mundane and repetitive tasks such as scheduling test programmes and analysing data. In some cases, there simply isn’t enough time to go through this process for all the design concepts, and potentially promising ideas have to be abandoned.
Perhaps more than any other sector, the automotive industry is experiencing these pressures at the moment. The incoming – and as yet unfinalised – Euro 7 regulations look set to add yet more complexity to ICE development at a time when most manufacturers are already diverting huge amounts of resources into electrification, which is itself a hugely complicated topic.
Artificial intelligence (AI) may hold the key to unpacking the vast data sets generated by these complex systems. It can speed up everyday tasks, automate processes and provide insight that might otherwise remain hidden.
“AI is about efficiency,” comments Gary Brotman, CEO of machine learning specialist Secondmind. “You’re trying to take repetitive tasks, automate them, and take that burden away from a human expert.”
The Cambridge-based company is part of the rich seam of computer science talent that has sprung up around the city’s famous university. It was this same leafy part of England, for instance, that gave us the natural language processing used in Amazon’s Alexa function.
Secondmind focuses on AI for automotive design and development. Its customers already include the Mazda Motor Corporation in Japan, where Secondmind’s optimisation engine is being used to accelerate the process of ECU calibration for hybrid and electric vehicles.
we certainly don’t sit in the doomsday world. Our aim is to be the ‘second mind’ of the engineer, providing them with tools to make them better at what they do and to shift their expertise to new challenges. There’s yet to be any case where I’ve seen where any new algorithms are going to replace 10 or 20 years of knowledge and experience
A modern combustion engine ECU can have well over 50,000 parameters, many of which interact with each other. Calibrating each one by hand becomes an impossible task, even if you have the resources to do so.
There are numerous practicalities to consider. For example, if you’re looking at cold start behaviour in a test vehicle, you only have a matter of moments before the engine warms up, after which it will need to be left for several hours to cool down again. Physical prototypes are also expensive to build and transport, and once you’ve shipped them to, say, a cold climate test there’s no guarantee the weather conditions will actually play ball.
Increasingly, vehicle manufacturers are embracing model-based calibration. Here, the powertrain and the sub systems within it are represented by a mathematical model, which can be tested in place of a physical prototype. As a result, there’s no vehicle preparation or logistics to consider, the tests can be simulated far quicker than real-time and numerous duplicates of the same model can be run in parallel.
But even with an automated approach, there’s simply too much data to run through every single combination of parameters. This is where AI comes in. The technology can do incredible things with large, complex data sets, but it also enables engineers to work smarter, reducing the amount of testing and data collection that’s involved.
“We think of big data – more sensors and more data points – as better for decision making if, say, we’re driving down the road in an autonomous vehicle, but data is also the foe in making design and development processes more efficient,” comments Brotman. “Less data is better for efficiency, whether you’re looking at the early stage design of the vehicle and the systems within it or hardware in the loop calibration. We use just 20 per cent of the data that would typically be required to solve the same problems.”
Secondmind’s Active Learning technology uses Bayesian optimisation to home in on the solution to a problem. This is effectively a filtering algorithm that directs the system towards the inputs that will yield a target output.
An example might be the goal of minimising brake specific fuel consumption for a given range of speed and load points, without breaching predefined limits for NOx emissions, cylinder pressure and turbo speed. A physics model for the relationship between these variables is generated in the cloud and used to run an experiment on the first set of values. The input conditions are automatically refined, based on these outputs, and the process iterates towards an optimal solution.
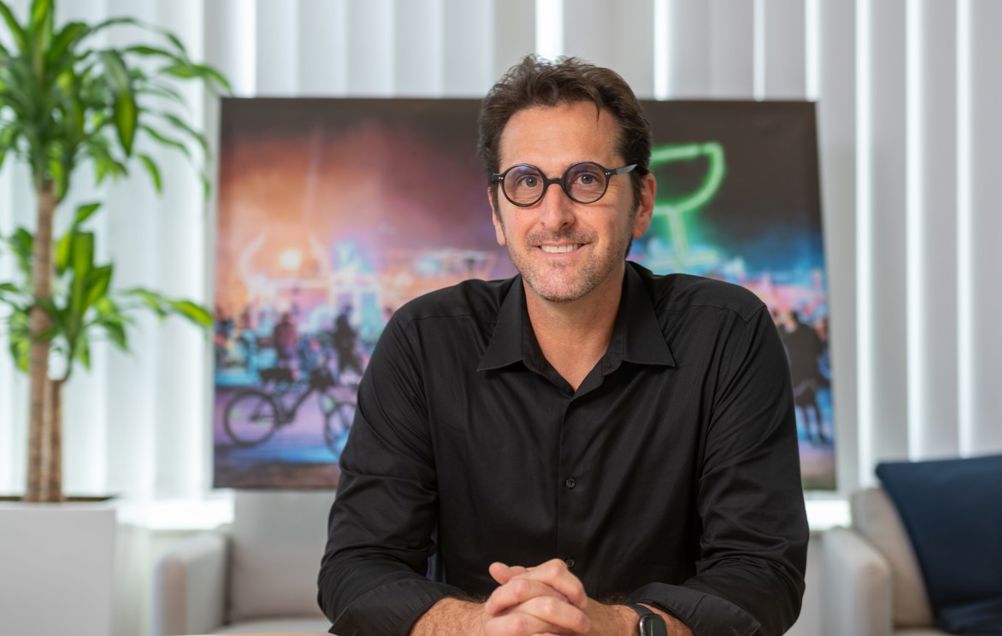
The end result is much the same as using traditional statistical methods in model-based calibration, but the solution can be achieved far more efficiently with intelligent optimisation. It can be carried out entirely virtually or blended with experimental data and the engineer’s own intuition.
Secondmind’s Optimization Engine is also in the early stages of helping engineers optimise the design of complex vehicle systems. It’s hoped that this will give them better configuration choices and a higher degree of confidence in the early stages of the design. The initial aim is not to eliminate physical testing completely, but rather to minimise the dependency on it, Brotman explains.
“This doesn’t guarantee that you’ll reach the optimum solution with no mistakes and no re-working – at least not yet” he comments. “But when it comes to hardware in the loop testing, the risk of having an error in the physical prototype will be far lower and the calibration process will be even faster. One OEM customer said that due to the expected reduction of wear and tear on the test bench, they would only have to fabricate three prototype engines in a future program rather than five.”
When it comes to calibration, Secondmind’s AI methods have so far been confined to optimising values within the ECU. As such, it’s populating maps and look up tables that work with conventional control algorithms. In time, it’s possible that it could also generate the code. A key point here is that the machine learning algorithms used by Secondmind operate on a transparent basis that can be tracked and monitored. This means a control algorithm generated with these techniques could, theoretically, be signed off with conventional safety tests, unlike the deep learning methods where it’s very hard to prove exactly what’s happening inside the system.
“I think there’s plenty of development yet to come with generative AI. We’re starting to see large language models that can write code. How efficient they can be at doing that remains to be seen, but things are moving quickly,” notes Brotman.
So, is there a danger that the human engineers could themselves be replaced by AI?
“As technology advances to do certain tasks, the human side of the equation will always be impacted in some way, shape or form,” replies Brotman. “The question is, what does the company do about it? Do they reskill? Do they get people to change jobs? There’s going to be an adaptation that will happen.
“But we certainly don’t sit in the doomsday world. Our aim is to be the ‘second mind’ of the engineer, providing them with tools to make them better at what they do and to shift their expertise to new challenges. There’s yet to be any case where I’ve seen where any new algorithms are going to replace 10 or 20 years of knowledge and experience – that will still be relevant in some way, even if you’re switching to a new field, like moving from ICE powertrains to fully electric.”
As vehicles continue to grow in complexity, the need for fast and efficient calibration methods will only increase. And it doesn’t stop at the point where the vehicle is released to the public; over the air (OTA) updates and the move towards a software-defined vehicle mean that continuous development for the life of the vehicle is entirely possible. All of which makes eliminating repetitive tasks and focusing on the bigger picture that little bit more important.
‘Archaic rules’ torn up to green light new nuclear
Lack of data about windpower being cheaper than nuclear was, I felt, the question that you replied to. and as the context is energy security it would...